Training Data for Self Driving Cars: Revolutionizing Software Development
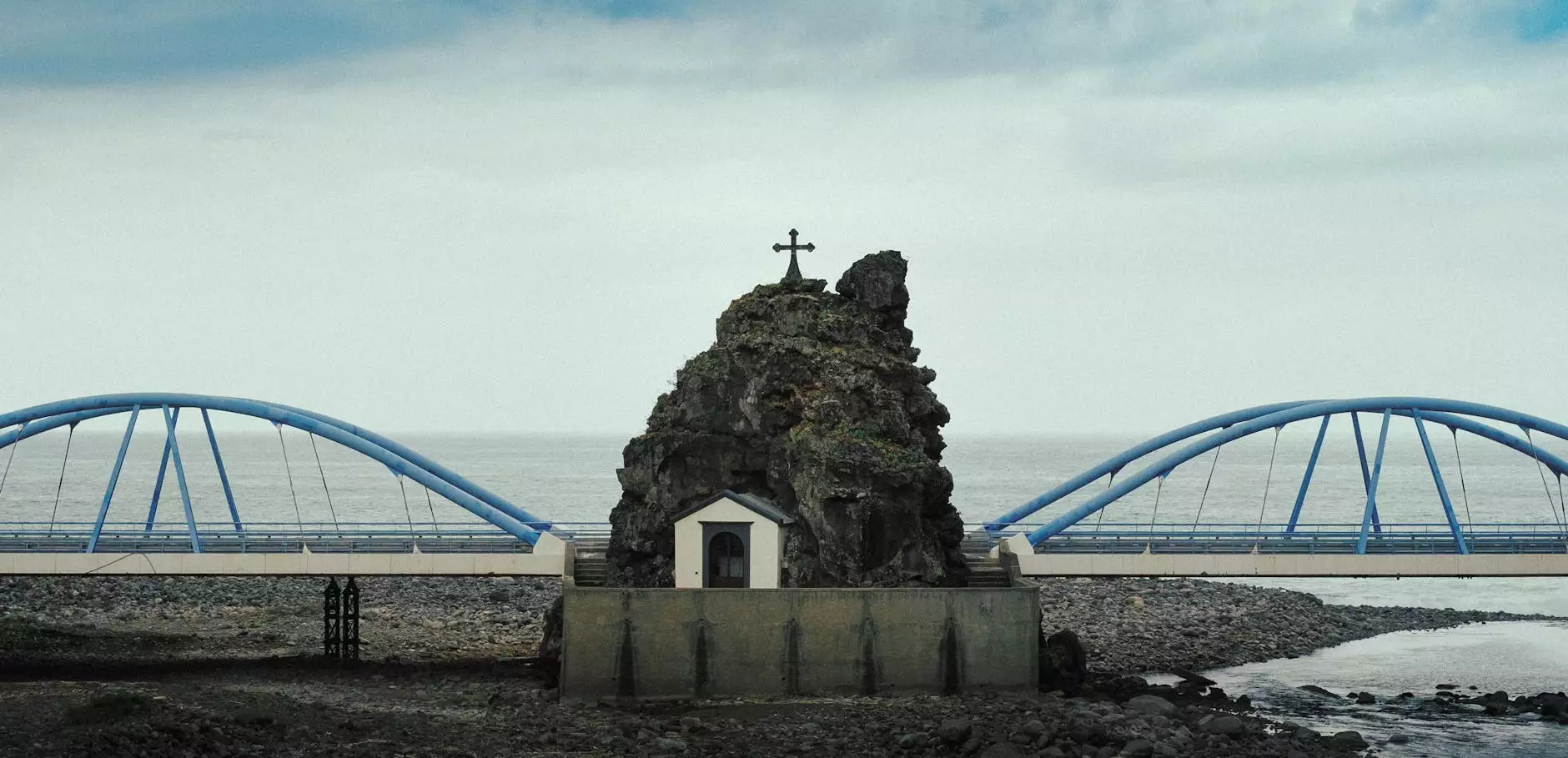
The automotive industry stands on the brink of a massive transformation, driven by innovative software development and advanced technologies. At the heart of this revolution is the role of training data for self driving cars, which is essential in designing algorithms that allow vehicles to navigate autonomously. In this article, we will delve deep into the significance of training data, how it’s utilized in self-driving technology, and how companies like KeyMakr are contributing to this evolving landscape.
Understanding Self-Driving Cars
Self-driving cars, or autonomous vehicles, possess the ability to operate without human guidance, relying instead on sophisticated algorithms and deep learning techniques. These systems use a combination of hardware, including sensors and cameras, and software that processes the information gathered from the environment to make real-time decisions.
The Role of Training Data
Training data serves as the backbone of the artificial intelligence models that power self-driving technologies. It refers to the vast amounts of data collected from various environments and situations that autonomous systems can encounter on the road. This data is crucial for training algorithms to recognize objects, interpret signals, and understand driving behaviors.
Types of Training Data
Self-driving cars require diverse and extensive datasets to ensure safety and efficiency. Here are some types of training data used in developing autonomous vehicles:
- Image Data: High-resolution images captured from various sensors help the car's AI recognize objects, pedestrians, traffic signs, and road conditions.
- Sensor Data: Data from LiDAR, radar, and ultrasound sensors are utilized to perceive the vehicle's immediate surroundings.
- Video Data: Continuous video feeds from cameras provide dynamic context to training models, allowing car systems to learn from live traffic scenarios.
- Weather Conditions: Training data must encompass every possible weather scenario – from rain to fog – to prepare autonomous systems for real-world driving conditions.
- Geographical Data: Detailed maps and information about various terrains and road types are essential for effective path planning and navigation.
Why Quality Training Data Matters
In software development for autonomous vehicles, the quality of training data is paramount. Inadequate or biased datasets can lead to inaccuracies in decision-making and could jeopardize passenger safety. Here are several reasons why quality training data is critical:
- Safety: Well-curated datasets ensure that self-driving cars can respond appropriately to scenarios, such as emergency braking when detecting a pedestrian.
- Performance: High-quality training data contributes to improved accuracy in object detection, path planning, and speed regulation.
- Adaptability: Diverse datasets help algorithms adapt to different driving conditions, enhancing vehicle reliability across various environments.
- Bias Reduction: A balanced dataset helps reduce potential biases, allowing autonomous vehicles to treat all road users fairly and safely.
Source and Collection of Training Data
The collection of training data for self driving cars involves various methods. Some key approaches include:
1. Real-World Driving
Developers often deploy vehicles equipped with sensors and cameras on public roads to gather real-time data. This method allows for the collection of valuable information regarding everyday driving scenarios and challenges.
2. Simulations
Simulation environments are vital for generating synthetic datasets. By creating virtual reality scenarios, developers can explore extreme conditions and rare events that might be difficult to encounter in real life.
3. Crowdsourced Data
Some companies employ crowdsourcing mechanisms to gather data from various users, enhancing the diversity of their datasets. This data can include travel patterns, road conditions, and user feedback on autonomous systems.
Challenges in Training Data Collection
While the importance of training data is clear, the process of collecting it presents several challenges:
- Data Privacy: Collecting real-world data raises privacy concerns among users, necessitating strict compliance with regulations.
- Environmental Variability: The unpredictability of road conditions and human behavior complicates data collection.
- Data Annotation: Accurately labeling data is time-consuming and requires expert knowledge to ensure high-quality training sets.
- Cost: The deployment of sensor-laden vehicles and the infrastructure required for data collection can be a financial burden.
The Future of Training Data in Autonomous Vehicles
As the landscape of automotive technology evolves, so will the methodologies for collecting and utilizing training data. Emerging trends to watch include:
1. Enhanced AI Algorithms
With advancements in machine learning techniques, algorithms will become increasingly efficient at processing larger datasets, enabling quicker training times and improved accuracy.
2. Improved Data Sharing
Collaboration among various automotive manufacturers and technology developers to share anonymized training data can lead to better, more comprehensive datasets.
3. Real-Time Learning
Future systems may integrate real-time learning capabilities, allowing vehicles to learn from new situations as they occur on the road, continuously improving their performance.
Key Contributors in Training Data Development
Several companies are pioneering efforts in the development of training data for self-driving cars. Companies like KeyMakr are at the forefront, providing specialized software solutions that assist businesses with data annotation and quality assurance.
KeyMakr: Innovating Training Data Solutions
KeyMakr stands out in the field of software development, particularly through its dedicated services focused on data quality and training data curation. KeyMakr strives to ensure that data used in autonomous systems is not only comprehensive but also meets the highest standards of accuracy and diversity.
By leveraging advanced methodologies and technologies for data collection and annotation, KeyMakr empowers automotive developers to stay ahead in this rapidly evolving marketplace.
Conclusion
In conclusion, the role of training data for self driving cars cannot be understated. As we transition towards a future dominated by autonomous vehicles, the demand for high-quality, accurate training data will only grow, propelling advancements in safety, efficiency, and adaptability. Through innovation and commitment to quality, companies like KeyMakr are positioning themselves as leaders in this transformative journey, ensuring that the promise of self-driving technology becomes a reality.