Revolutionizing Software Development with Auto-Annotate Technology
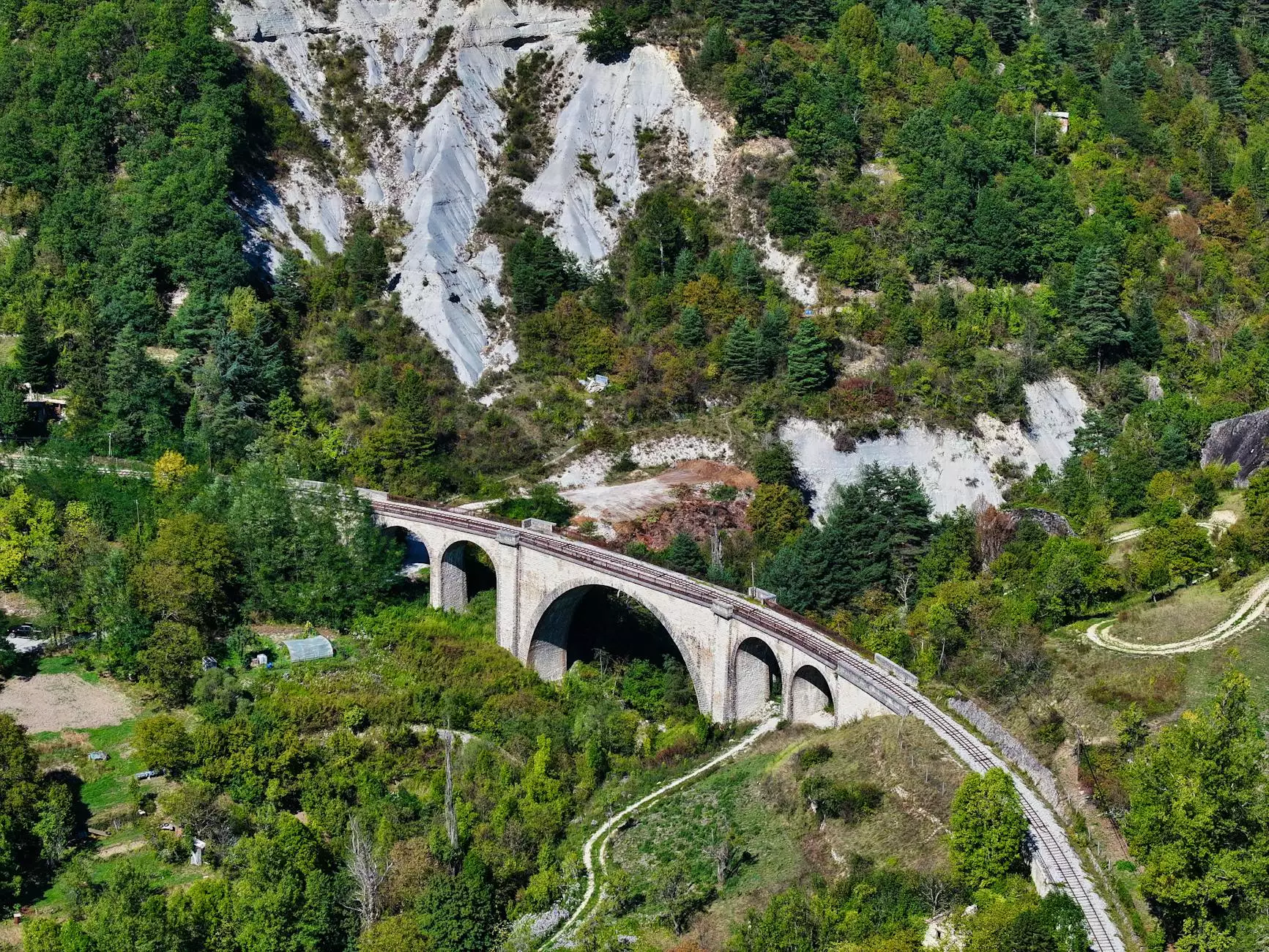
In the rapidly evolving landscape of technology, staying ahead of the curve is imperative for businesses looking to thrive. One of the most significant advancements in software development is the introduction of auto-annotate technology, which automates the process of data labeling, significantly enhancing efficiency and accuracy. This article delves into how auto-annotate is reshaping the software development industry and why it’s essential for businesses to embrace this innovation.
Understanding Auto-Annotate Technology
Auto-annotate refers to the automated process of labeling and tagging data sets using machine learning algorithms and artificial intelligence. This technology simplifies the cumbersome task of data annotation, which is essential for training machine learning models, particularly in fields such as image recognition, natural language processing, and computer vision.
The Importance of Data Annotation
Data annotation is a critical step in the machine learning pipeline. It involves labeling data so that machine learning models can learn from it. Without accurately annotated data, models can produce unreliable results, leading to poor business decisions. Traditional manual annotation processes are often time-consuming, costly, and prone to human errors.
How Auto-Annotate Works
At its core, auto-annotate employs sophisticated algorithms to identify patterns in data, automatically generating labels for various data points. This involves several steps:
- Data Collection: Gather a substantial amount of data relevant to the task at hand.
- Model Training: Use a small, manually annotated data set to train the model.
- Automated Annotation: Once trained, the model can predict labels for new, unannotated data.
- Quality Assurance: Implement validation steps to ensure the accuracy of the auto-generated annotations.
Benefits of Auto-Annotate Technology
1. Increased Efficiency
The most significant advantage of auto-annotate technology is the drastic reduction in time needed for data annotation. Instead of spending weeks or months manually labeling data, businesses can use automated systems to accomplish the same tasks in a fraction of the time.
2. Cost-Effective Solutions
By minimizing the manual labor associated with data annotation, companies can significantly reduce operational costs. Auto-annotate enables businesses to allocate resources more effectively, focusing on high-value tasks that require human intelligence.
3. Enhanced Accuracy
Humans are prone to errors, especially in repetitive tasks like data labeling. Auto-annotate technology mitigates this risk by using advanced algorithms that can maintain high levels of consistency and precision in labeling, leading to better model performance.
4. Scalability
As businesses grow, so does their data. Manually scaling annotation processes can be daunting. Auto-annotate allows for seamless scaling, enabling businesses to handle vast amounts of data without compromising on quality.
Real-World Applications of Auto-Annotate Technology
The versatility of auto-annotate technology allows it to be applied across various industries. Below are some real-world applications:
1. Healthcare
In the healthcare sector, auto-annotate can streamline the process of annotating medical images, such as X-rays, MRIs, and CT scans. This automation helps in training diagnostic AI models that can assist doctors in identifying diseases more accurately and quickly.
2. Autonomous Vehicles
For autonomous vehicles, accurate data labeling is crucial for the AI systems to interpret the surrounding environments correctly. By using auto-annotate, companies can efficiently label vast amounts of driving data, which accelerates the development of safer self-driving technologies.
3. E-commerce
In e-commerce, auto-annotate technology can be employed to categorize and tag product images and descriptions efficiently. This automated process enhances searchability and improves user experience by providing customers with relevant product recommendations.
Implementing Auto-Annotate in Your Business
Integrating auto-annotate technology into your software development process requires careful planning and execution. Here are some essential steps to consider:
1. Assess Your Needs
Before adopting auto-annotate, evaluate your data annotation needs. Determine the volume of data, the complexity of the annotations, and the desired outcomes for your machine learning models.
2. Choose the Right Tools
There are various tools and platforms available that provide auto-annotate technology. It’s crucial to select a solution that aligns with your specific requirements. Look for features such as scalability, customization options, and ease of integration into existing workflows.
3. Train Your Team
While auto-annotate technology automates many processes, having a team that understands the underlying principles is vital. Provide training on how to use the tools effectively and how to validate the automatically generated annotations.
4. Monitor and Evaluate
After implementation, continuously monitor the performance of your auto-annotate system. Regularly evaluate the accuracy of the annotations and make adjustments as necessary to enhance model training.
Challenges and Considerations
While auto-annotate technology offers numerous benefits, it’s important to consider potential challenges:
1. Quality Control
Maintaining the quality of auto-generated annotations is critical. Implementing a robust quality control process to verify the accuracy of annotations is essential to prevent introducing biases into your models.
2. Data Privacy and Security
When handling sensitive data, ensuring compliance with data protection regulations is paramount. Make sure your auto-annotate solutions adhere to legal standards regarding data privacy.
3. Dependence on Quality Input Data
The accuracy of auto-annotate technology heavily relies on the quality of the input data. If the training data is flawed, the outcomes will be as well. It's crucial to invest time and resources into gathering quality data for training your models.
The Future of Auto-Annotate Technology
As technological advancements continue to unfold, the future of auto-annotate technology looks promising. With continuous improvements in algorithms and machine learning capabilities, we can expect:
1. Greater Accuracy
Future advancements will likely lead to even more sophisticated algorithms capable of delivering highly accurate annotations across diverse datasets.
2. Enhanced Customization
The ability to customize auto-annotation processes will become more refined, allowing businesses to tailor the technology to their specific needs and workflows.
3. Wider Adoption Across Industries
As more organizations recognize the benefits of auto-annotate, its adoption will continue to broaden across various industries, transforming how businesses handle data.
Conclusion
In conclusion, auto-annotate technology represents a paradigm shift in the software development field, offering unparalleled efficiency, cost savings, and accuracy in data annotation. As businesses continuously strive to optimize their processes, embracing this technology is not just an option; it is a necessity to remain competitive in today’s data-driven landscape. By understanding its applications, benefits, and implementation strategies, organizations can harness the power of auto-annotate to drive innovation and success.